Mapping all hedges in France, using high resolution images
Blog > Article > Mapping all hedges in France, using high resolution images
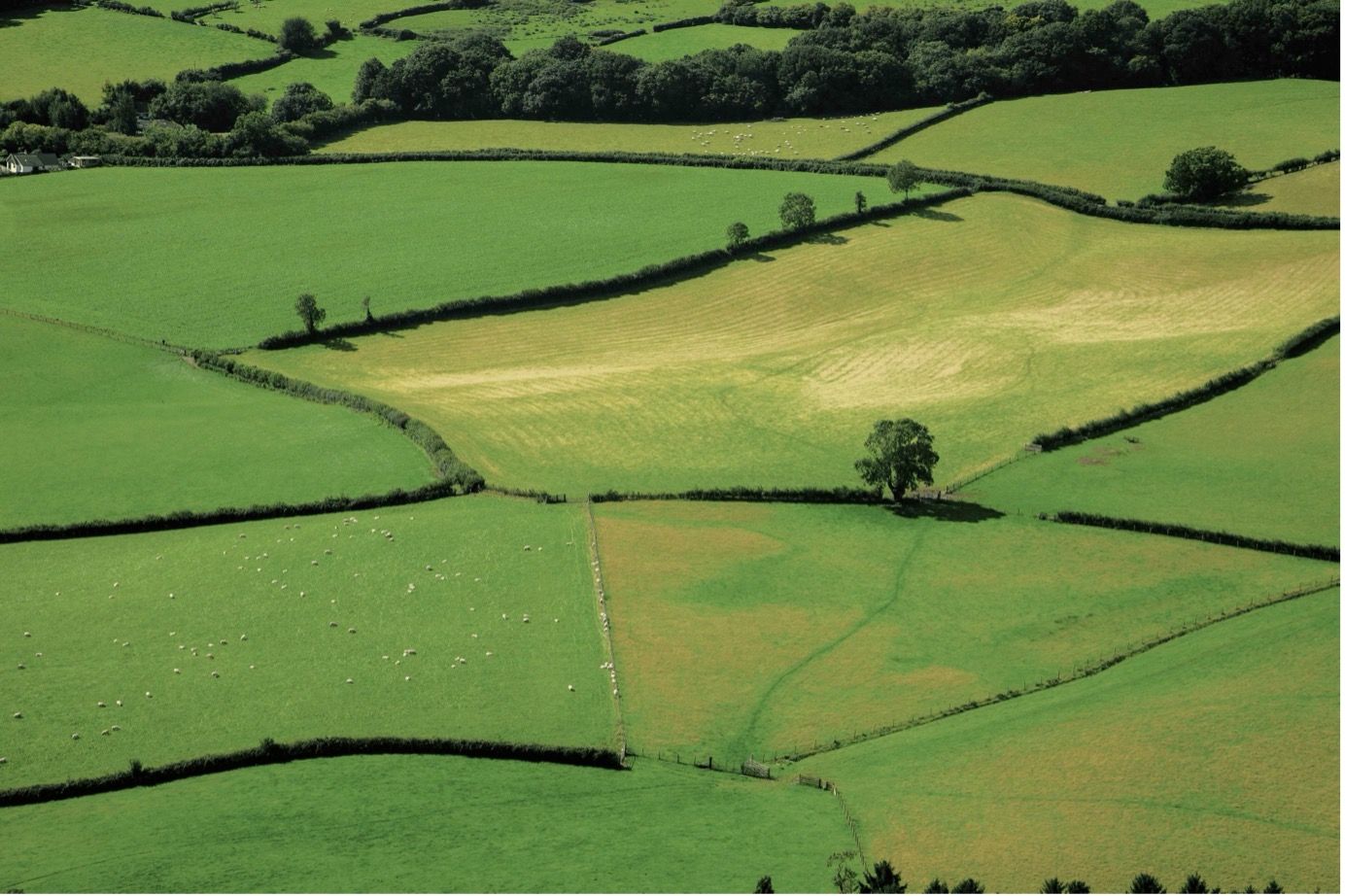
Understanding the spatial distribution and temporal changes of landscape features such as hedges is crucial. Using high resolution images, we mapped all of them.
Understanding the spatial distribution and temporal changes of landscape features such as hedges is crucial. Using high resolution images, we mapped all of them.
Understanding the evolution of hedges is crucial for municipalities and provinces. These natural structures play a significant role in carbon sequestration, biodiversity preservation, and the ecological integrity of rural and semi-urban landscapes. While accurate and up-to-date data on hedge networks remain elusive, Meteory has managed to develop a solution that maps all hedges from 2008 until this day for Fance.
The imperative for advanced hedge analytics
Hedges are not merely landscape features; they are dynamic ecosystems that contribute to: • Carbon Storage: They act as carbon sinks, mitigating greenhouse gas emissions. • Biodiversity: Hedges serve as habitats and corridors for various species, promoting ecological diversity and resilience. • Soil Conservation: They prevent soil erosion and promote hydrological stability. • Agricultural Benefits: Hedges can enhance crop yields by protecting against wind and supporting beneficial insects.
Given these benefits, the need for precise and regularly updated data is clear.
The Challenge of Monitoring Hedges
Traditionally, territories have relied on tools like ALDO and the BD TOPO, a comprehensive topographic database from the French National Institute of Geographic and Forest Information (IGN), to estimate and map the presence of hedges. While these resources provide foundational data, they have some limitations: • Infrequent Updates: These datasets are not regularly refreshed, making it difficult to track changes over time or respond to environmental crises promptly. • Inconsistent Detail : The level of detail can be insufficient for fine-grained ecological analysis and conservation planning. • Limited Scope: Existing tools may not capture all physical and biological characteristics of hedges, such as species diversity, health, and structural integrity, which are vital for ecological assessments.
There is an opportunity to revolutionize and refine how we monitor and understand these critical natural resources. Thanks to the incredible work done by the IGN with the aerial imagery of the BD ORTHO, taking high resolution pictures of the entire country on a regular basis, a great new data source was provided to further work on.
Our approach to hedge detection using high-resolution aerial imagery and advanced models
We have developed a model to monitor hedges across France that uses aerial imagery as input to infer the shapes of hedges present. Our model accomplishes this through two broad steps.
Machine learning based hedge classification
The initial step of our approach is an in-house developed Neural Network (CNN) model that is trained using the previously mentioned BD ORTHO dataset as input and an extensive manually labeled training dataset for validation.
To ensure that our neural network gives reliable results we apply specific training data weighing and resampling, which accounts for the fact that most images contain far fewer hedges than they contain other things.
The resulting neural network can convert aerial images into images which depict the probability of each pixel being a hedge.
Hedges Line Synthesis
As visible in the image above, the model decides which parts of the image are likely to be hedges, but having only these per pixel probabilities is still far away from having the direct shapes of the hedges to enable qualitative and quantitative analysis.
The second step in our model is thus to synthesise the lines out of the probability prediction from our neural network. This synthesis is accomplished through the following processing steps:
- We firstly apply a blur filter to the image to smooth out the distribution of probabilities.
- We apply a threshold. All probabilities above a certain threshold are considered to be pixels of hedges. All other pixels are not hedges.
- Finally, we filter out forests and urban areas. The neural network sometimes misinterprets parts of forests as hedges. These areas are filtered out of the hedges we consider. We likewise filter out hedges in urban areas.
4.The processed images are then converted into polygon vectors and the small irregular ones are filtered out
5.Since the polygons were generated from images, their shapes are rather rough. To achieve a more natural shape, we simplify the geometry of the vectors, then create lines from them and clean the few irregularities automatically.
Using these results, our partners are able to monitor how many hedges they have and how they have been evolving over time. They can see where they lost some as well as where they gained some, giving a comprehensive view of the territory.
New solutions for new challenges
The ability to monitor hedges effectively addresses a significant gap in environmental management. By leveraging high-resolution aerial imagery and advanced object detection techniques, our model provides critical data that is not only more accurate but also more timely than traditional methods.
This innovation not only enhances our understanding of ecological dynamics but also empowers stakeholders to make informed decisions that support environmental sustainability and resilience. The ongoing development and refinement of such models, facilitated by open-source datasets and community-driven data initiatives, underscore the vital role of technology in advancing our ecological and conservation goals.
If you want to know the state of your hedges on your territory, contact us at contact@meteory.eu, we will make it happen.
Pierre Blanchet • June 3, 2024
Co-founder of Meteory